Machine learning pipeline for direct detection experiments
After painstakingly thorough work, I am pleased to see our new paper, led by Andre Scaffidi, out today as part of the DARWIN direct dark matter detection collaboration! 👏
We use a semi-supervised anomaly detection pipeline, comprising of a variational autoencoder trained on simulated background events and a classifier distinguishing between electronic and nuclear recoils, to demonstrate new physics searches at future direct detection experiments – here focusing on DARWIN. This enables us to look for `anomalous signals’ (ie, unexpected under the background simulations) in a physics-independent manner.
Our approach improves sensitivity with respect to a likelihood-based method, while remaining model-agnostic.
Check out the paper here: https://lnkd.in/dv-f5NDB
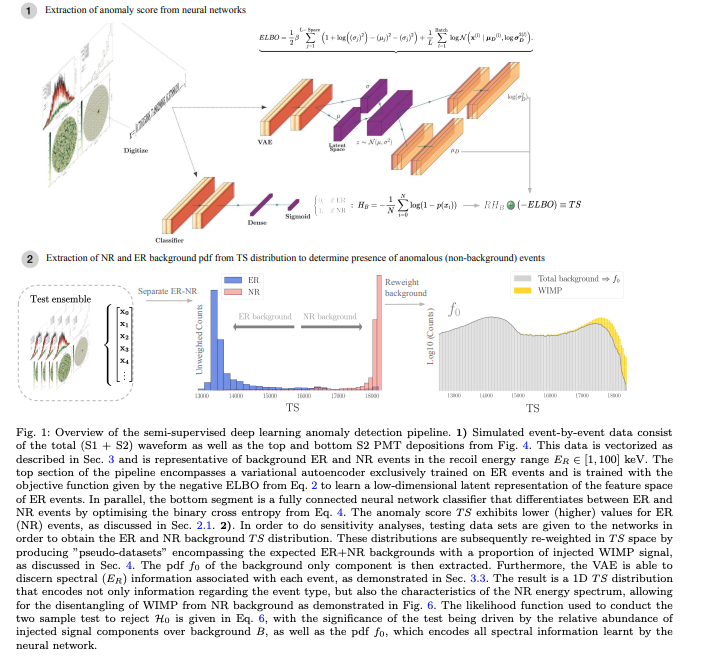